Making Predictions With Data And Python
- 30.12.2022
- 40
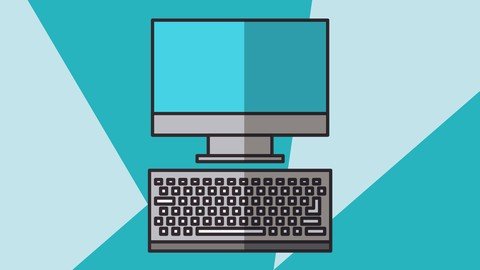
Last updated 12/2018
MP4 | Video: h264, 1280x720 | Audio: AAC, 44.1 KHz
Language: English | Size: 563.29 MB | Duration: 4h 11m
Build Awesome Predictive Models with Python
Build Awesome Predictive Models with Python
What you'll learn
Understand the main concepts and principles of Predictive Analytics and how to use them when building real-world predictive models.
Properly use scikit-learn, the main Python library for Predictive Analytics and Machine Learning.
Learn the types of Predictive Analytics problem and how to apply the main models and algorithms to solve real world problems.
Build, evaluate, and interpret classification and regression models on real-world datasets.
Understand Regression and Classification
Refresh your visualization skills
Requirements
Knowledge of the Python programming language is assumed. Basic familiarity with Python's Data Science Stack would be useful, although a brief review is given. Familiarity with basic mathematics and statistical concepts is also advantageous to take full advantage of this course.
Description
Python has become one of any data scientist's favorite tools for doing Predictive Analytics. In this hands-on course, you will learn how to build predictive models with Python.
During the course, we will talk about the most important theoretical concepts that are essential when building predictive models for real-world problems. The main tool used in this course is scikit -learn, which is recognized as a great tool: it has a great variety of models, many useful routines, and a consistent interface that makes it easy to use. All the topics are taught using practical examples and throughout the course, we build many models using real-world datasets.
By the end of this course, you will learn the various techniques in making predictions about bankruptcy and identifying spam text messages and then use our knowledge to create a credit card using a linear model for classification along with logistic regression.
About the author
Alvaro Fuentes is a Data Scientist with an M.S. in Quantitative Economics and a M.S. in Applied Mathematics with more than 10 years of experience in analytical roles. He worked in the Central Bank of Guatemala as an Economic Analyst, building models for economic and financial data. He founded Quant Company to provide consulting and training services in Data Science topics and has been a consultant for many projects in fields such as; Business, Education, Psychology and Mass Media. He also has taught many (online and in-site) courses to students from around the world in topics like Data Science, Mathematics, Statistics, R programming and Python.
Alvaro Fuentes is a big Python fan and has been working with Python for about 4 years and uses it routinely for analyzing data and producing predictions. He also has used it in a couple of software projects. He is also a big R fan, and doesn't like the controversy between what is the "best" R or Python, he uses them both. He is also very interested in the Spark approach to Big Data, and likes the way it simplifies complicated
things. He is not a software engineer or a developer but is generally interested in web technologies.
He also has technical skills in R programming, Spark, SQL (PostgreSQL), MS Excel, machine learning, statistical analysis, econometrics, mathematical modeling.
Predictive Analytics is a topic in which he has both professional and teaching experience. Having solved practical problems in his consulting practice using the Python tools for predictive analytics and the topics of predictive analytics are part of a more general course on Data Science with Python that he teaches online.
Overview
Section 1: The Tools for Doing Predictive Analytics with Python
Lecture 1 The Course Overview
Lecture 2 The Anaconda Distribution
Lecture 3 The Jupyter Notebook
Lecture 4 NumPy - The Foundation for Scientific Computing
Lecture 5 Using Pandas for Analyzing Data
Section 2: Visualization Refresher
Lecture 6 Plotting with Matplotlib
Lecture 7 Visualizing data with Pandas
Lecture 8 Statistical Visualization with Seaborn
Section 3: Concepts in Predictive Analytics
Lecture 9 What Is Predictive Analytics?
Lecture 10 How to Do Predictive Analytics?
Lecture 11 Machine Learning - Supervised Versus Unsupervised Learning
Lecture 12 Supervised Learning - Regression and Classification
Lecture 13 Models and Algorithms
Section 4: Regression: Concepts and Models
Lecture 14 scikit-learn
Lecture 15 The Multiple Regression Model
Lecture 16 K-Nearest Neighbors for Regression
Lecture 17 Lasso Regression
Lecture 18 Model Evaluation for Regression
Section 5: Regression: Predicting Crime, Stock Prices, and Post Popularity
Lecture 19 Predicting Diamond Prices
Lecture 20 Predicting Crime in US Communities
Lecture 21 Predicting Post Popularity
Section 6: Classification: Concepts and Models
Lecture 22 Logistic Regression
Lecture 23 Classification Trees
Lecture 24 Naive Bayes Classifiers
Lecture 25 Model Evaluation for Classification
Section 7: Classification: Predicting Bankruptcy, Credit Default, and Spam Text Messages
Lecture 26 Predicting Credit Card Default
Lecture 27 Predicting Bankruptcy
Lecture 28 Building a Spam Classifier
Lecture 29 Further Topics in Predictive Analytics
The course is designed for Data analysts or data scientists interested in learning how to use Python to perform Predictive Analytics as well as Business analysts/business Intelligence experts who would like to go from descriptive analysis to predictive analysis. Software engineers and developers interested in producing predictions via Python will also benefit from the course.
https://www.udemy.com/course/making-predictions-with-data-and-python/
Download From 1DL
DOWNLOAD FROM 1DL.NET
Download ( Rapidgator )
DOWNLOAD FROM RAPIDGATOR.NET
Download (Uploadgig)
DOWNLOAD FROM UPLOADGIG.COM
Download ( NitroFlare )
DOWNLOAD FROM NITROFLARE.COM
Please Help Me Click Connect Icon Below Here and Share News to Social Network | Thanks you !